In today’s rapidly evolving technological landscape, IT teams grapple with the complexities of managing hybrid environments, where on-premises infrastructure coexists with cloud-based services. A report by 451 Research highlights the prevalence of this challenge, revealing that over 60% of organizations operate in hybrid environments. Yet, many struggle to manage the intricacies of this architecture effectively.
Legacy monitoring tools are not designed to quickly provide visibility into on-premises and cloud infrastructure. This lack of visibility in hybrid environments makes identifying and resolving issues difficult. Compounding these challenges, businesses struggle to correlate the overflow of raw data from disparate sources, quickly turning this information into a liability rather than an asset. But what if there was a way to quickly cut through the noise and transform that data into actionable insights, providing a holistic view of the entire IT ecosystem?
Enter hybrid observability powered by AI—an approach that empowers IT operations teams to overcome alert fatigue, pinpoint root causes faster, and proactively manage infrastructure complexities.
What is hybrid observability?
Think of hybrid observability as the GPS for your IT infrastructure. It maps the health of systems on-premises and across multiple clouds, collecting and analyzing data from events, metrics, logs, and traces. This comprehensive view helps teams proactively identify issues, resolve incidents quickly, optimize resource use, and ensure seamless user experiences.
Overcoming the challenges of hybrid observability with real-world examples
While hybrid observability offers insights into IT environments, real-world implementation presents unique challenges. Observability solutions enhanced by AI meet these challenges, improving IT infrastructure management.
Challenge 1: Too much data, not enough insight
The problem: IT teams are inundated with data from various sources—logs, metrics, and traces. Amidst this data deluge, identifying critical issues becomes like finding a needle in a haystack.
The AI-powered solution: AI-powered observability platforms leverage machine learning (ML) to establish both static and dynamic thresholds for various metrics. Static thresholds are predefined limits, while dynamic thresholds adapt based on historical data and patterns. ML algorithms continuously analyze incoming data, identifying anomalies that breach these thresholds and correlating them with other events to pinpoint root causes. This not only surfaces the most critical alerts but also significantly reduces alert fatigue, allowing IT teams to focus on resolving high-impact issues and strategic initiatives.
A real-world example: The Minnesota Vikings leveraged LogicMonitor’s LM Envision platform to manage their complex IT environment for business and football operations. AI-powered anomaly detection and root cause analysis allowed them to proactively identify and resolve issues, ensuring smooth gameday experiences and efficient business operations.
Challenge 2: Adapting to dynamic infrastructures
The problem: Cloud components in hybrid environments scale up and down rapidly, leaving traditional monitoring tools struggling to keep pace. It’s like trying to track constantly shifting traffic patterns without a reliable map. This lack of visibility then creates blind spots and makes it harder to manage resources and identify potential bottlenecks proactively.
The AI-powered solution: Automated, event-based discovery mechanisms continuously monitor the cloud environment for changes, automatically identifying new resources as they are provisioned and de-provisioned. By leveraging this real-time visibility, IT teams can apply AI-powered predictive analytics to forecast potential issues even with fleeting resources, ensuring uninterrupted service and optimal performance.
A real-world example: Schneider Electric used LogicMonitor to achieve significant efficiency gains through AI-driven anomaly detection and dynamic thresholds. This proactive approach reduced alert fatigue and allowed them to focus on critical issues, ultimately improving operational efficiency. They reduced the time needed to identify and resolve issues and decreased the number of alerts by about 40%, from 17,000 to 10,000.
Challenge 3: Legacy systems and skill gaps
The problem: Many organizations rely on outdated on-premises systems that aren’t built for modern observability. Integrating them with cloud-native tools is like fitting square pegs into round holes. IT teams may also lack the skills to manage these new tools effectively.
The AI-powered solution: Improved collaboration through a shared platform with intuitive interfaces and automated workflows empowers teams with diverse skill sets to work together seamlessly. AI-driven automation reduces the need for manual intervention and lowers the barrier to entry for adopting new tools and practices.
A real-world example: Henrico IT successfully integrated their legacy systems with LogicMonitor, enabling them to monitor critical infrastructure components and optimize network connectivity. The platform’s intuitive interface and automated workflows empowered their team, regardless of skill level, to manage their hybrid environment effectively.
Challenge 4: Organizational silos and resistance to change
The problem: Organizational silos, where different teams are responsible for different parts of the infrastructure, can hinder communication and collaboration. Resistance to change is another common obstacle, as IT staff may hesitate to abandon familiar workflows for new, unfamiliar tools and practices. The lack of transparent governance and ownership over observability initiatives can hinder progress.
The AI-powered solution: Cost optimization through AI-driven resource management provides a tangible incentive for change by identifying underutilized assets, right-sizing cloud resources, and maximizing ROI. The shared platform and collaborative features of hybrid observability tools also foster communication and break down silos, enabling a more cohesive approach to IT management.
A real-world example: John Holland broke down silos and fostered collaboration across teams by implementing LM Envision. This cohesive approach improved problem-solving and reduced operational impacts, leading to a 40% cost reduction and a 75% reduction in IT monitoring tools.
Challenge 5: Cloud costs, compliance, and incident response
The problem: Managing cloud costs and ensuring compliance can feel like walking a tightrope. One wrong move and organizations could face skyrocketing expenses or regulatory penalties. Plus, incident response in a hybrid environment is like juggling multiple balls—all it takes is one drop to cause chaos.
The AI-powered solution: Hybrid observability platforms provide insights into resource utilization and optimization opportunities, helping IT teams confidently walk that tightrope. They automate data collection and reporting for compliance, while AI-driven incident response tools analyze complex incidents and suggest remediation steps, reducing downtime and minimizing the risk of compliance breaches.
A real-world example: Hain Celestial Group faced challenges managing its cloud costs and ensuring compliance in its hybrid IT environment. By implementing LogicMonitor’s cloud monitoring solution, they were able to gain real-time visibility into their cloud spending, identify areas for optimization, and ensure compliance with industry regulations. This resulted in over 60% cost savings in monthly IT expenditures. Additionally, LogicMonitor’s logging capabilities streamlined troubleshooting, turning complex multi-call issues into efficient single-call resolutions.
By addressing these challenges head-on, AI-powered hybrid observability enables organizations to maximize the full potential of their hybrid IT environments. It allows them to achieve greater efficiency, resilience, and agility, ultimately contributing to business success in today’s digital landscape.
How AI-powered observability platforms work under the hood
Underpinning the AI-powered solutions mentioned above is a sophisticated data processing pipeline that enables these platforms to ingest, analyze, and derive insights from the vast amounts of data generated by hybrid environments.
Data collection: Think of the platform as a gatherer of information from different sources. It collects data from APIs, agents installed on servers or containers, and log shippers that centralize logs for analysis. This data comes in various formats, such as metrics (e.g., CPU usage), logs (event records), and traces (details of request flow within applications).
Data processing: Next, the platform processes this diverse data. It normalizes and transforms it into a unified format for consistent analysis. This involves storing data in specialized databases like time-series databases for metrics, log aggregation systems for logs, and graph databases for understanding relationships between different components.
Data analysis: Here’s where the magic happens. The platform’s AI engine continuously analyzes the data for anomalies, traces the root causes of issues, and predicts future behavior. It does this in real-time and batch modes, providing immediate insights and deeper analysis of historical data.
Visualization and alerting: The platform then presents the analyzed data in user-friendly formats, such as customizable dashboards, reports, and alerts. These visualizations help IT teams and stakeholders quickly understand system health and performance, enabling proactive incident response.
Furthermore, advanced platforms often incorporate explainable AI, providing transparency into the decision-making processes of their models to foster trust and understanding. This transparency can involve highlighting the key factors influencing an anomaly or prediction. Additionally, to maintain accuracy and effectiveness as IT environments evolve, AI models require continuous training and updates, ensuring they adapt to new technologies and changing usage patterns.
How to select the right AI-powered hybrid observability platform
Choosing the ideal AI-powered hybrid observability platform is a critical decision that can significantly impact your organization’s IT efficiency and overall performance. Here’s a structured approach to help you navigate the selection process:
- Define your requirements
- Scope: Determine the breadth of your IT environment (on-premises, cloud, hybrid) and the specific technologies you need to monitor.
- Scalability: Choose a platform that can grow with your evolving infrastructure and accommodate increasing data volumes.
- Integration: Ensure seamless integration with your existing tools and workflows to avoid disruptions and maximize efficiency.
- AI capabilities: Identify the specific AI features most valuable to your organization (e.g., anomaly detection, root cause analysis, predictive analytics).
- Evaluate vendor options
- Experience and expertise: Look for vendors with a proven track record in observability, AI, and a strong commitment to security.
- Platform maturity: Assess the platform’s maturity, features, user interface, and ease of use. Ensure it aligns with your organization’s specific security requirements and compliance standards.
- Security features: Inquire about built-in security features such as encryption (at rest and in transit), role-based access controls, vulnerability scanning, threat detection, and incident response capabilities.
- Compliance: Verify that the platform adheres to relevant industry regulations and compliance standards (e.g., GDPR, HIPAA) to protect sensitive data.
- Customer support: Confirm the availability of comprehensive customer support, including security-specific resources and training, to facilitate smooth adoption and ongoing utilization.
- Prioritize key features
- End-to-end visibility: Choose a platform that consolidates data from diverse sources into a single pane of glass for holistic monitoring, ensuring comprehensive coverage and cloud-native monitoring capabilities.
- Real-time monitoring: Ensure real-time data collection and analysis for proactive issue detection and resolution.
- Customizable dashboards: Select a platform that allows you to tailor dashboards to your specific needs and preferences.
- Alerting and incident management: Opt for robust alerting mechanisms and incident management workflows to streamline response and resolution.
- Cost-effectiveness: Consider the total cost of ownership, including licensing, implementation, training, and ongoing maintenance.
How to ensure seamless implementation
To ensure a smooth transition, the adoption of a phased implementation strategy is key. Start with a pilot program or focus on critical areas of your infrastructure. Emphasize change management with clear communication and comprehensive training for your team.
Remember, selecting an AI-powered hybrid observability platform is not a one-size-fits-all process. Take the time to thoroughly assess your organization’s unique needs and evaluate vendors against those specific requirements. By following this approach and prioritizing key features, you can confidently choose a platform that empowers your IT team, optimizes operations, and drives business success.
The future of IT operations: Proactive and intelligent
AI-powered hybrid observability is changing IT operations by introducing proactive and intelligent monitoring as the new standard. This transformation will redefine how businesses approach IT infrastructure management as more organizations use the power of AI to predict issues before they arise, continuously optimize system performance, and ensure seamless digital experiences for their users.
As automation becomes integral to IT operations, the role of human intervention will evolve towards strategic oversight and innovation. The future of IT monitoring lies in AI-driven insights and automation, which will revolutionize operational efficiency, minimize downtime, and build resilient IT infrastructures that drive business success.
Ready to transform your IT operations through Hybrid Observability powered by AI?
Don’t get bogged down building fragmented solutions or juggling multiple vendors. You need a single, AI-powered platform that simplifies hybrid observability, delivers actionable insights across your entire IT estate, and accelerates your time to value.
LogicMonitor’s platform unites deep infrastructure monitoring expertise with intelligent automation, giving you the power to tame complexity, predict and prevent issues, and optimize both performance and costs. Experience the difference of a holistic, AI-driven approach to observability.
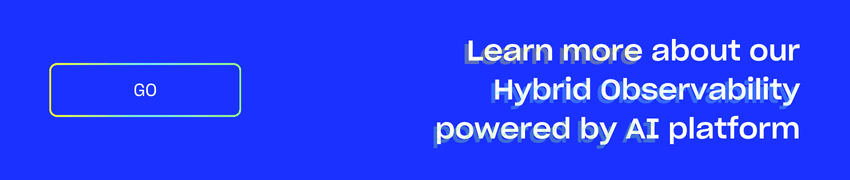
Subscribe to our blog
Get articles like this delivered straight to your inbox